Regulatory Compliance and Transparency in Artificial Intelligence
The concept of transparency in artificial intelligence has been debated extensively. Transparent AI allows humans to understand the conclusions being drawn from AI algorithms. Black-Box AI lacks transparency in that the conclusions drawn from algorithms are not explainable to humans. From a business perspective, proponents of black-box AI argue that requiring transparency in AI will limit the adoption of more accurate technology and that explainability should not be necessary for solutions that increase profits or reduce expenses. Proponents of transparency argue that AI systems should be able to both learn and explain their learning at the same time and to the extent that systems are drawing conclusions from data it is not unreasonable to expect them to document the basis for those conclusions. Generally, there is a perception that when it comes to AI, there is a trade off between accuracy and explainability which we believe can be expressed with the following diagram.
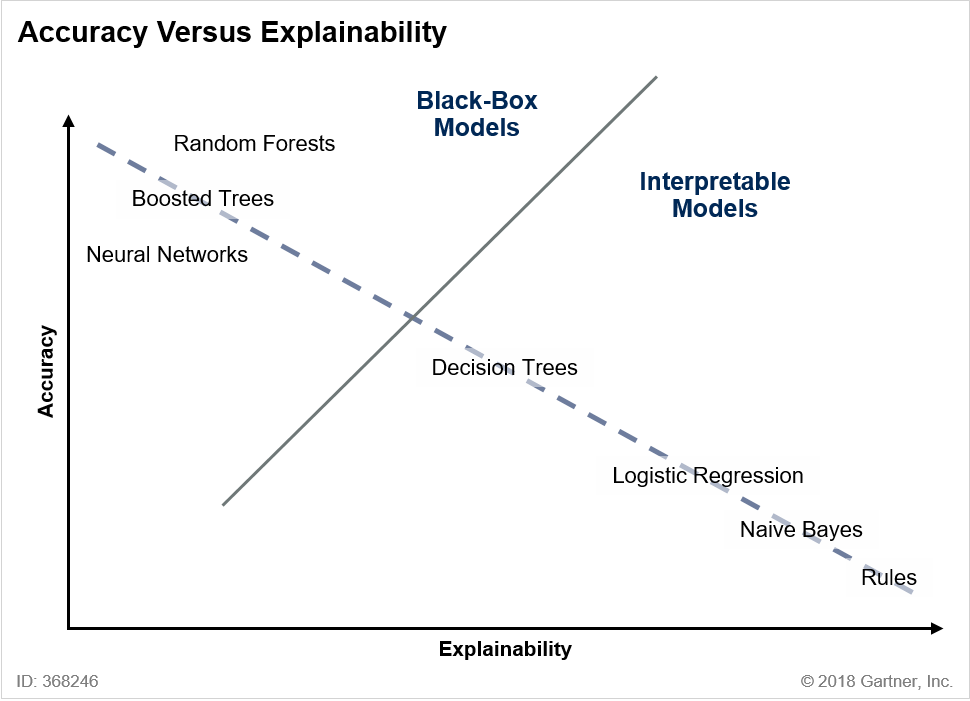
Source: Gartner “Build Trust With Business Users by Moving Toward Explainable AI,” Saniye Alaybeyi, et al, 12 October 2018
I am a technology attorney focusing on software including AI. From a legal perspective, I think transparency should be required for all projects that have a regulatory compliance or legal liability risk associated with it. Virtually every business in the US is subject to industry regulations directly effecting use of IT and sharing, using, and processing customer information. In addition, there are rules prohibiting discrimination and even one law that outright prohibits the use of profiling using automated means. In addition, many business decisions carry legal risks and we never know exactly what business records could become evidence in a court case. Legally, I am concerned about AI systems without sufficient transparency to provide the necessary evidence to defend a regulatory compliance investigation or a civil suit. For example, if you use an AI system to help evaluate mortgage applications, you should require the vendor to help you demonstrate that the conclusions drawn are not based upon race for example. If black-box solutions were used for this purpose and a later review of the approved loans showed a disparate impact on minorities, such a case would be extremely difficult to defend in court without evidence of exactly how the system evaluated each application.
Similarly, if you are using AI systems to process HR applications, you are going to want to be able to prove that the systems were not biased in a way that leads to discrimination in hiring claims. If you are subject to GDPR, you are going to need to demonstrate that your AI systems are not impermissibly profiling in violation of Article 22. In the last 10 years there has been a regulatory explosion in IT. It has become the norm for IT systems to be auditable. AI solutions deployed in businesses need to be transparent to the extent necessary to demonstrate compliance with applicable regulations and provide counsel the evidence necessary to defend civil litigation.
To the extent that black-box models are chosen for accuracy purposes, use of such models should be limited to non-regulated data sets where there is also a low risk of litigation arising from the actions taken in reliance on the model.